Nära samarbete med industri och näringsliv bidrar till att vår forskarutbildning alltid är aktuell och relevant.
Forskarutbildning
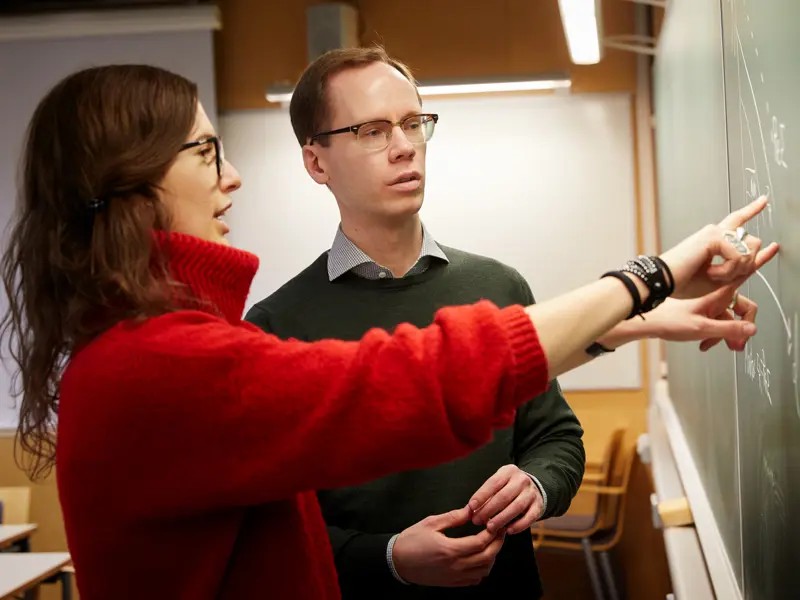
Doktorandkurser
En forskarutbildning är en kombination av individuell forskning, kurser och undervisning. Som doktorand väljer du aktiviteter med hjälp av din handledare och utifrån studieplanen i din forskarskola.
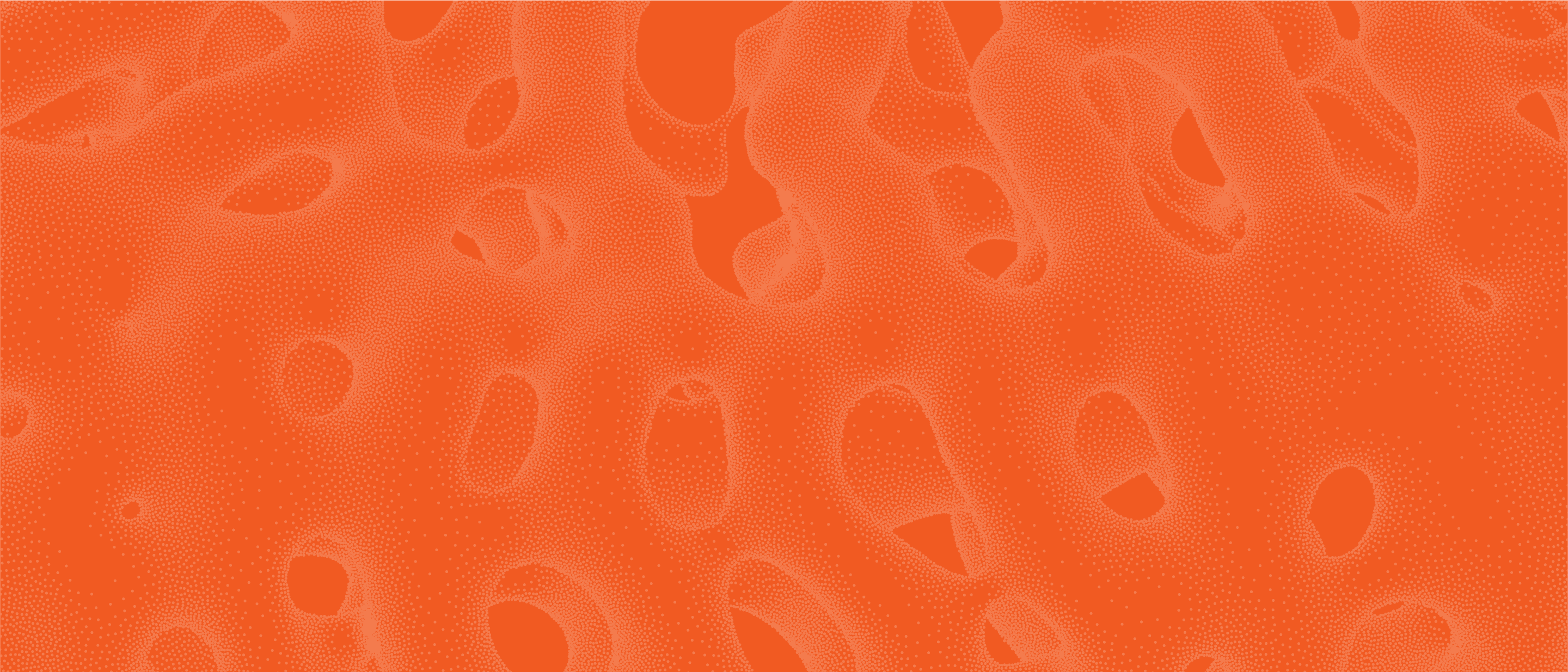
Doktorandtjänster
Som doktorand är du anställd av Chalmers. Alla doktorandtjänster annonseras under lediga tjänster.
Lediga tjänster