Vid institutionen för elektroteknik, E2, bedrivs forskning och utbildning inom områdena kommunikation, antenner och optiska nätverk, system- och reglerteknik, signalbehandling och medicinsk teknik samt elkraftteknik.
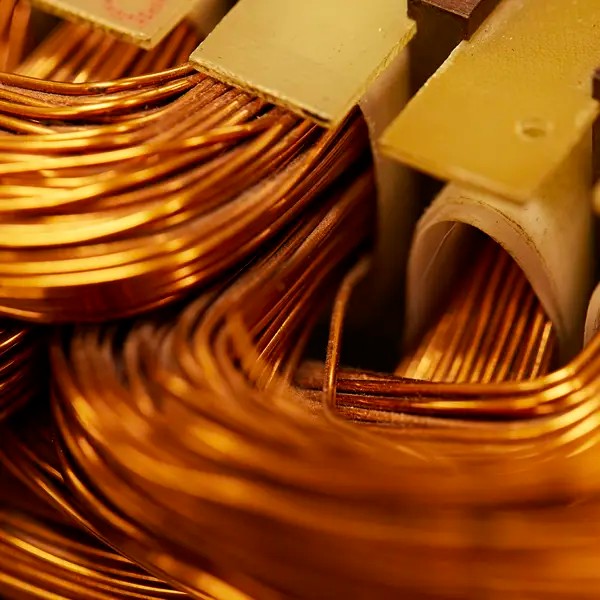
Forskning vid Elektroteknik
Institutionen för elektroteknik består av fyra forskningsavdelningar: Elkraftteknik, Kommunikation, antenner och optiska nätverk, Signalbehandling och medicinteknik samt System- och reglerteknik. Vi arbetar med hållbara och smarta lösningar på samhällsutmaningar
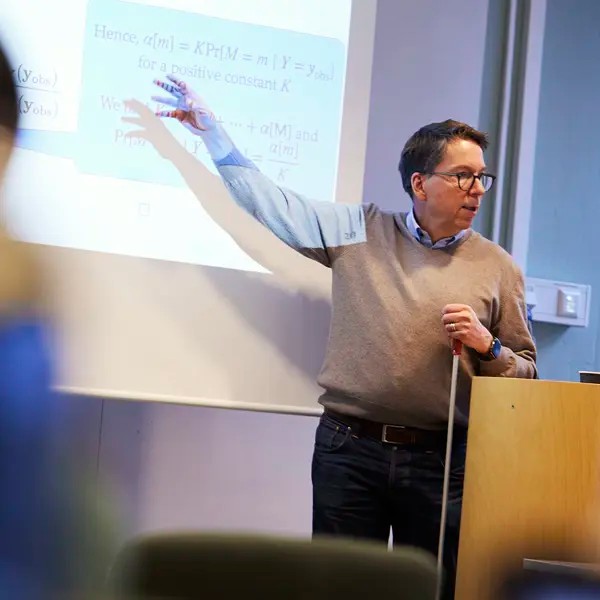
Utbildning vid Elektroteknik
Institutionen för Elektroteknik bedriver utbildning på många olika nivåer. Vi är involverade i flera kandidatprogram och ansvariga för masterprogrammen Biomedical Engineering, Communication Engineering, Electric Power Engineering och Systems, Control and Mechatronics. Du kan även läsa forskarutbildningen med ett brett utbud av kurser.