Vid institutionen för elektroteknik, E2, bedrivs forskning och utbildning inom områdena kommunikation, antenner och optiska nätverk, system- och reglerteknik, signalbehandling och medicinsk teknik samt elkraftteknik.
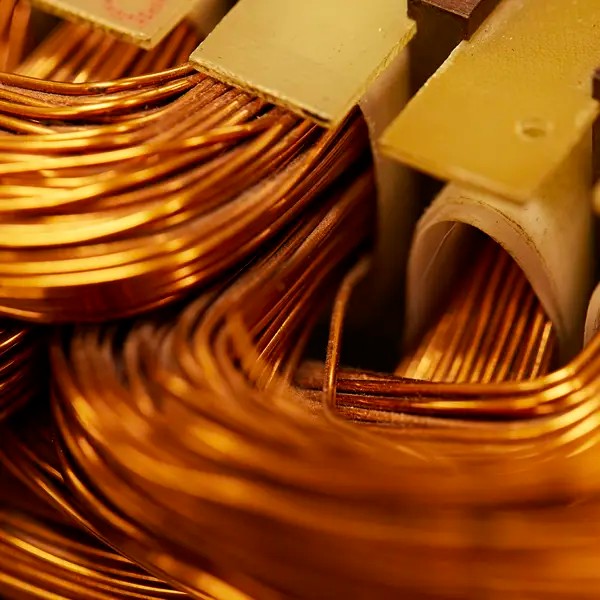
Forskning vid Elektroteknik
Institutionen för elektroteknik består av fyra forskningsavdelningar: Elkraftteknik, Kommunikation, antenner och optiska nätverk, Signalbehandling och medicinteknik samt System- och reglerteknik. Vi arbetar med hållbara och smarta lösningar på samhällsutmaningar
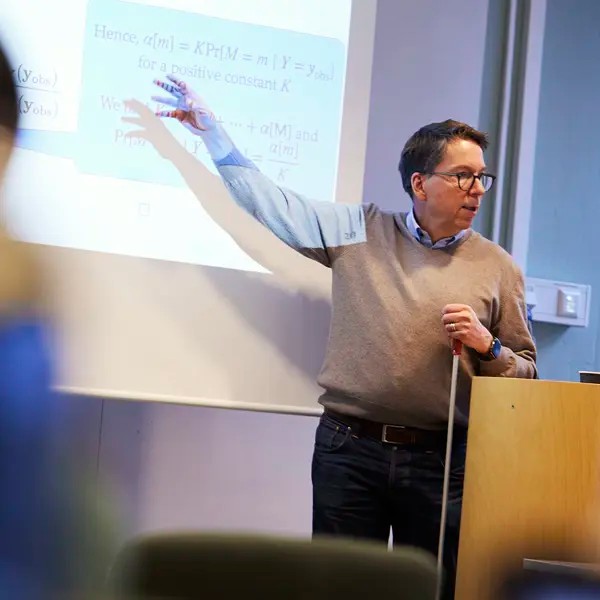
Utbildning vid Elektroteknik
Institutionen för Elektroteknik bedriver utbildning på många olika nivåer. Vi är involverade i flera kandidatprogram och ansvariga för masterprogrammen Biomedical Engineering, Communication Engineering, Electric Power Engineering och Systems, Control and Mechatronics. Du kan även läsa forskarutbildningen med ett brett utbud av kurser. Skrolla ned för att läsa mer om masterprogram och forskarutbildning.