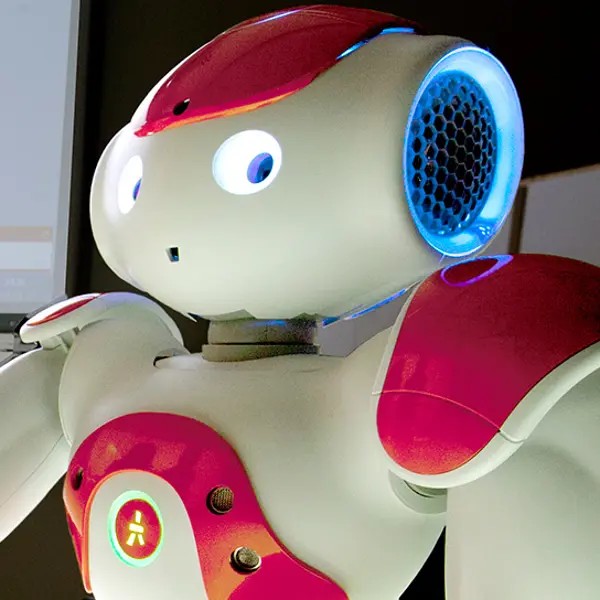
Världsledande forskning
Vår forskning ligger i internationell framkant och vi har mottagit flera prestigefulla anslag.
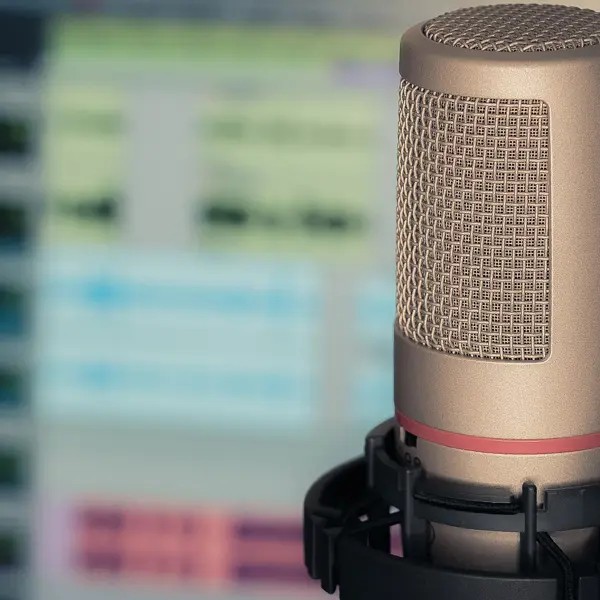
Möt våra forskare
Vilka utmaningar och möjligheter för dagens och framtidens digitala samhälle med sig? Hör våra forskare berätta
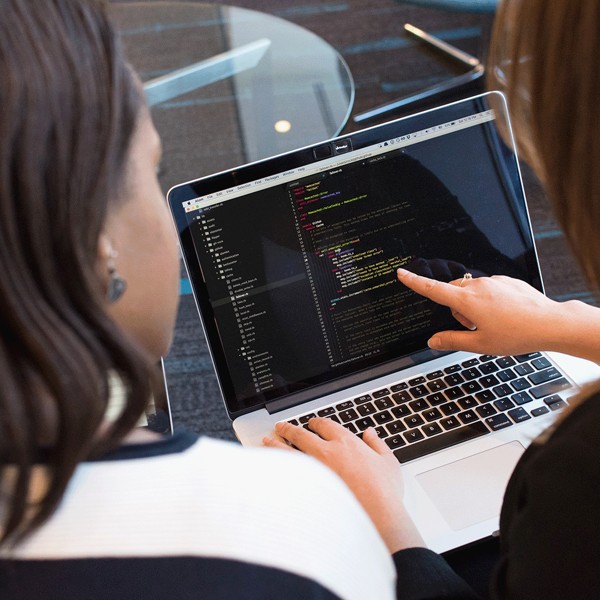
Vår utbildning
Bli en del av morgondagens samhälle och får möjlighet att vara med och forma den teknik som omger oss.
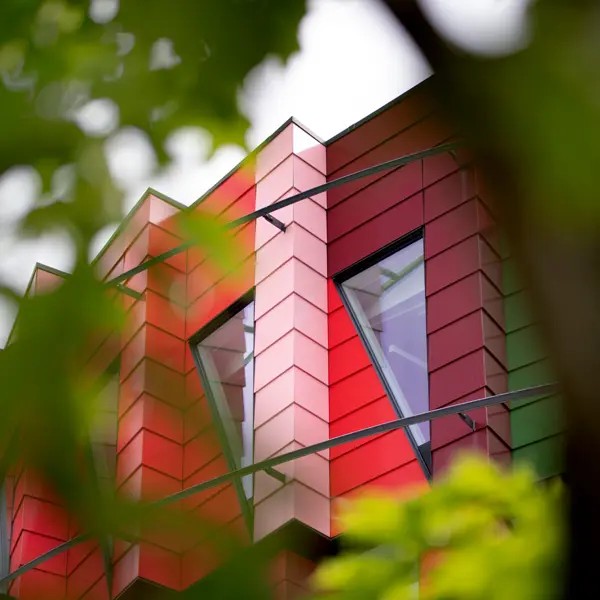
Det bästa av två världar
Att vara en delad institution mellan Chalmers och Göteborgs universitet ger oss unika möjligheter.