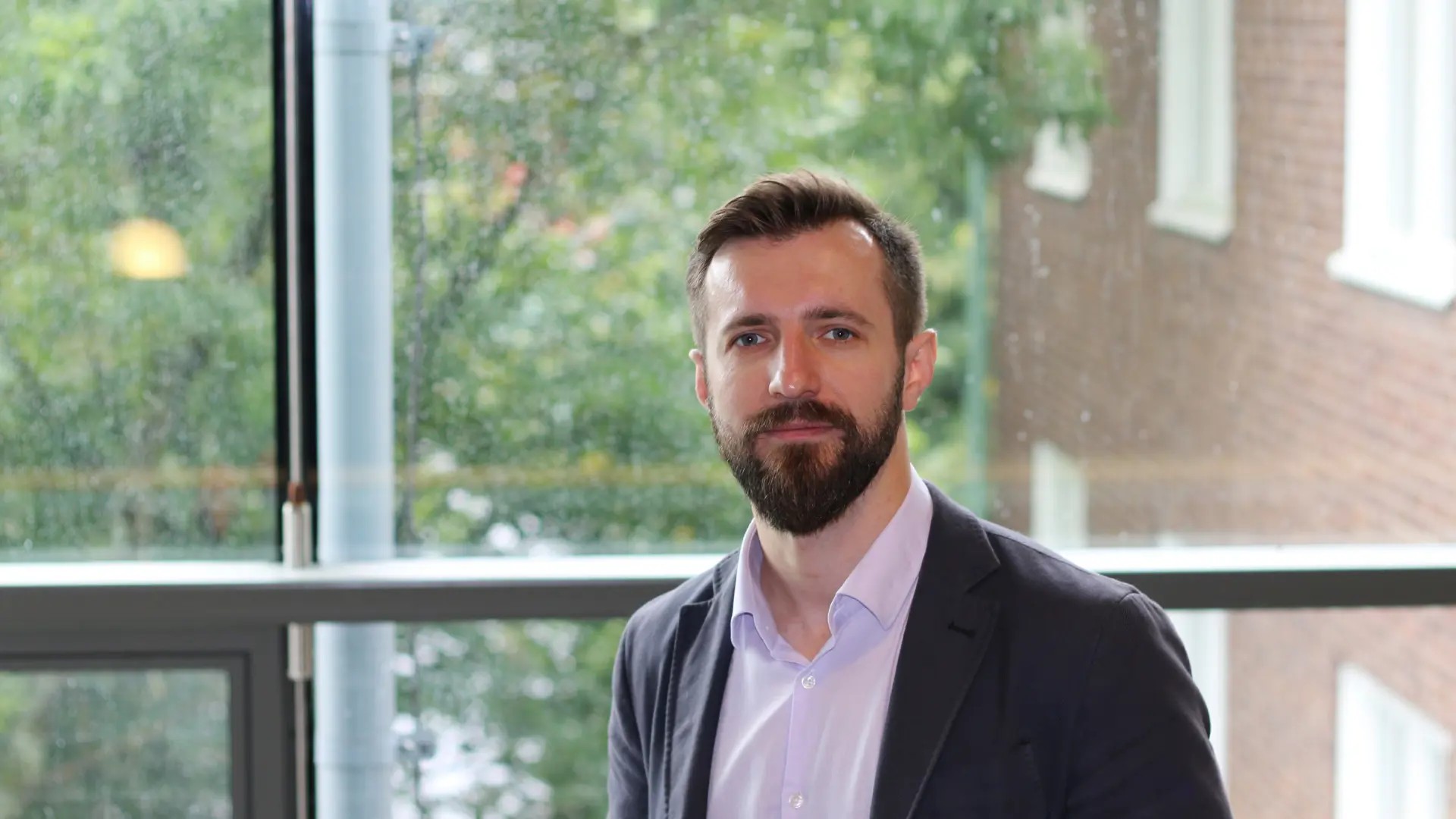
The understanding and effective manipulation of biological systems, i.e., the ability to predict the behaviour of biological systems enables designing of highly efficient therapies against disease and engineering of microbial processes that can shift us from a fossil to sustainable society. Towards this, we couple computer and biological sciences by bridging state-of-the-art machine learning, bioinformatics, mathematical modelling together with experimental techniques to advance systems biology research.
Our focus falls under the umbrella of three main research directions:
• Regulation and control of gene expression
• AI-driven synthetic biology for genome and protein engineering
• Exploring the planet’s enzymatic repertoire for bioremediation
More information can be found at the Zelezniak Lab's website
Regulation and control of gene expression
Starting from DNA, individual cells express proteins that regulate processes performing multiple reactions, ultimately resulting in the emergent behaviour of the entire organism.
Each of the steps in the molecular dogma hierarchy involves information transfer. For instance, whether a cell expresses some genes at few while other genes at thousands copy numbers to a significant extent is encoded directly in DNA.
In metabolic enzymes, amino acid sequence defines structural constraints of substrate specificity, reaction rate and stability of proteins. We are applying state-of-the-art and developing novel machine learning approaches to understand how evolution encoded information in biological sequences towards drawing quantitative genotype-phenotype maps.
AI-driven synthetic biology for genome and protein Engineering
Taking inspiration from a famous Fenman’s “What I cannot create, I do not understand”, using rules and patterns learned from natural systems, we apply artificial intelligence to create novel biological molecules with specific properties. For instance, by learning fundamental principles of gene expression DNA, we employ AI to construct new synthetic DNA molecules with defined gene expression levels.
We apply and develop novel machine-learning approaches to create synthetic gene expression systems and proteins with engineered, enhanced properties for biotechnology applications.
Exploring the planet’s enzymatic repertoire for bioremediation
Environmental pollutants such as plastics are serious ecotoxicological and health concerns globally. Bioremediation technologies utilising microorganisms and enzymes have the cost-effective and eco-friendly potential for combating environmental pollution.
Developing successful bioremediation technology requires understanding microbial metabolism, identification of critical enzymes, genes regulating dynamics, and composition of microbial communities.
Here, we are utilising our computational and high-throughput experimental technologies for the discovery, engineering, and practical testing of plastic degrading enzymes.
Our research in the news
- The Guardian: Bugs across globe are evolving to eat plastic, study finds
- National Geographic, France: Bactéries mangeuses de plastique: une bonne nouvelle pour la planète?
- Dagens Nyheter: Studie: Mikroorganismer lär sig bryta ner plast
- Forskning.se: Generna dirigerar både molekyl och mängd