At the Department of Life Sciences, LIFE, we explore how biological systems and innovative technologies can be used to convert biomass into foods, pharmaceuticals, materials, chemicals and fuels. We develop and apply advanced experimental and computational technologies to discover how biomolecules, living cells and organisms function and interact. We pioneer new methods for the prediction, prevention, diagnostics and treatment of diseases.
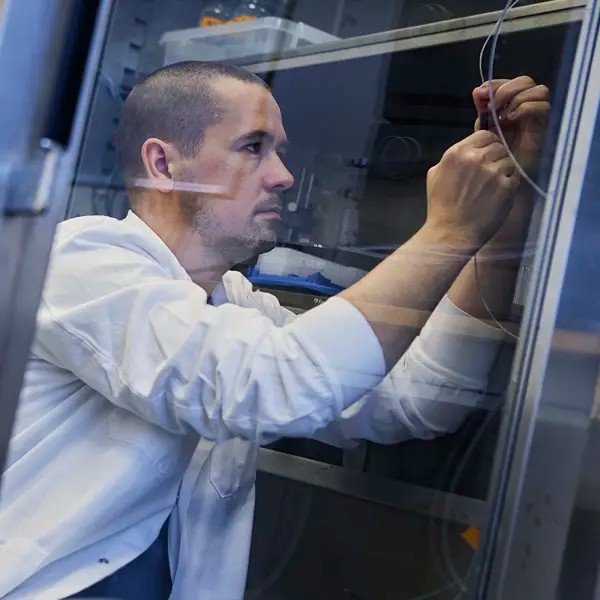
Our research
Research and innovation at LIFE is performed in close collaboration with academia, industry, and society at large.
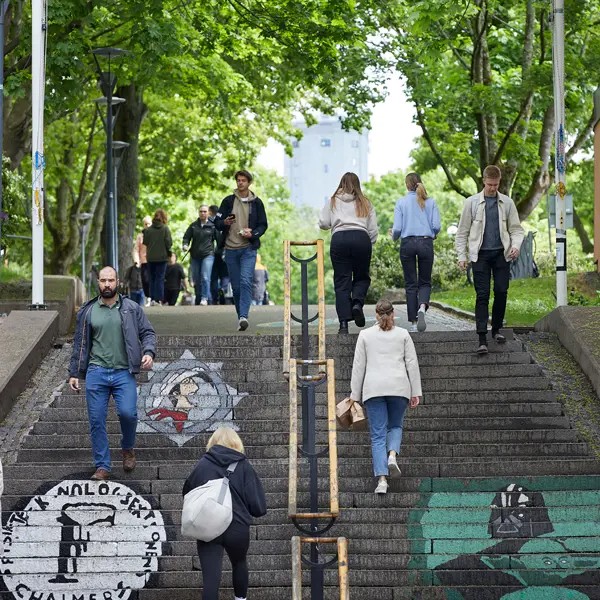
Impact in society
We work with a long-term perspective to create impact in the form of increased knowledge, innovations and collaboration.
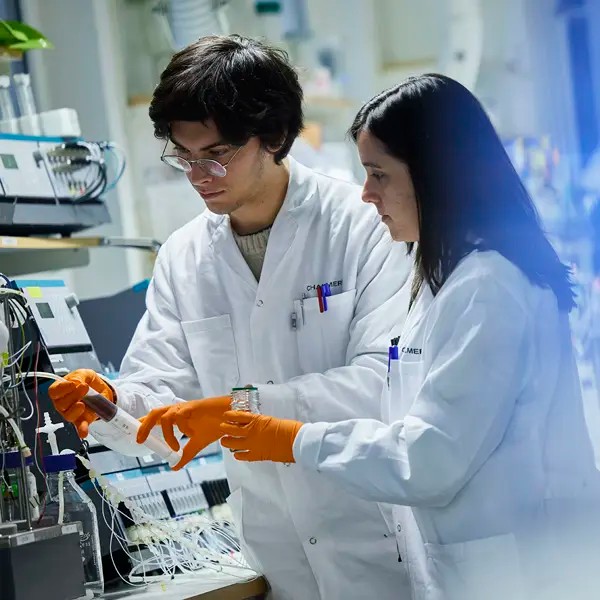
Master's thesis projects
Are you interested in doing a Master's thesis project at LIFE? Check out our available projects at the Thesis Portal.