Mathematical sciences investigate the fundamental concepts and laws that shape our way of thinking – from abstract structures to concrete models of reality. Mathematics is central to natural science and technology, but also plays an important role in fields such as social sciences, economics, and medicine. It is a research discipline in its own right, with distinct theories, methods, and questions. Basic research in mathematics and statistics is crucial for future innovations – often long before they are visible in practice.
The Department of Mathematical Sciences is a joint department of the University of Gothenburg and Chalmers University of Technology. It offers a strong and dynamic environment where research, education, and societal collaboration meet.
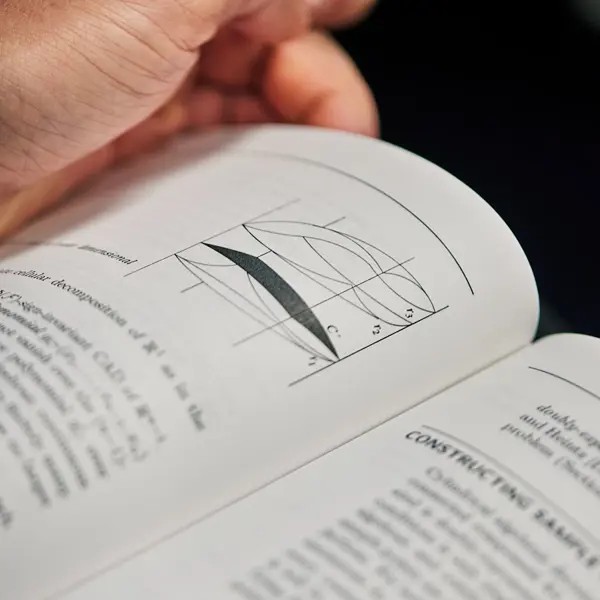
Our research
Read more about current research areas at Mathematical Sciences.

Our education
Read more about studying mathematics in Gothenburg.

Utilization
We collaborate with academia, business and society.

Seminar series
We hold regular seminars in most of our research areas.
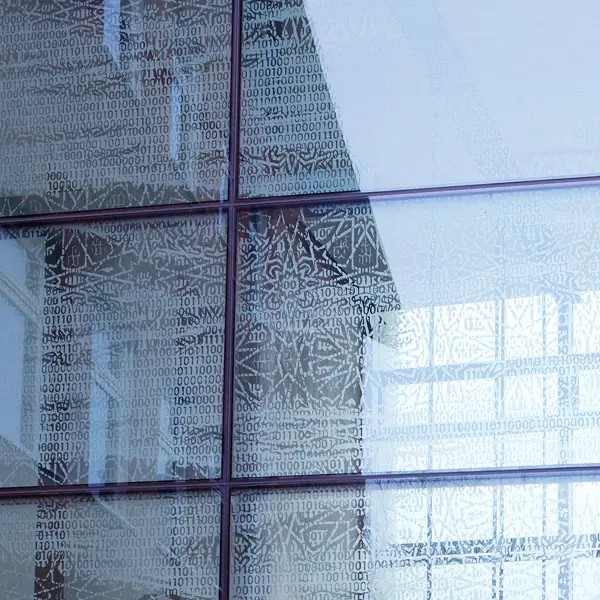
New employees at Mathematical Sciences
A presentation of our newly employed colleagues.
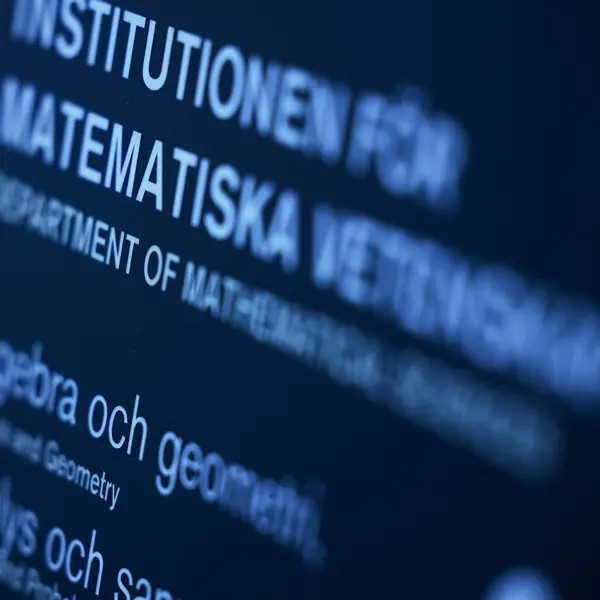
Guests at Mathematical Sciences
Scientific collaboration with colleagues from near and far is an important part of our research.