
Increasingly stricter regulations on emissions from lean burn engines, such as the Euro 7 standard, are approaching. This requires the development of catalytic materials that can reduce the toxic nitrogen oxides efficiently, at low temperatures. Researchers at the Department of Physics at Chalmers University of Technology, together with industrial partner Umicore, now present a study showing how machine learning could help engines run cleaner.
Catalytic converters reduce the amount of toxic pollutants emitted into the air from a vehicle’s exhaust system. Stricter regulations on emissions standards within the coming years, such as the European Union’s proposed Euro 7, aim at further reducing air pollution from vehicles. Therefore, improved catalysts are needed to limit the emissions of harmful pollutants.
The main technology of selective catalytic reduction of nitrogen oxides uses ammonia as a reducing agent. Thus, the catalytic material should promote the formation of a nitrogen–nitrogen bond between nitrogen oxides and ammonia in an oxygen-rich environment and prevent unwanted reactions, which include the oxidation of ammonia to even more nitrogen oxides or nitrous oxide.
Zeolites a powerful material for catalysts
One material with high activity for the selective catalytic reduction reaction is the chabazite zeolite promoted by copper. Zeolites are hydrated crystalline aluminosilicate consisting of small channels and cages, and copper is present as singly or doubly charged copper ions.
The copper-exchanged chabazite material has turned out to be a highly dynamic material. The copper ions are solvated by ammonia during typical low-temperature conditions forming mobile charged ammonia–copper–ammonia complexes that float in the zeolite channels and cages. The mobility of the complexes is crucial for the performance of the catalyst as two complexes in the same zeolite cage are needed for the reaction to proceed.
Have developed a machine learning forcefield
"Because of the highly dynamic character of the catalyst, computational investigations are important to understand how detailed structure and composition influence performance,” says Henrik Grönbeck, Professor at the Department of Physics at Chalmers University of Technology.
“In our recent study we have developed a machine learning forcefield – a computational model that is used to describe the forces between atoms. Our forcefield include long-range electrostatic interactions, which makes it possible to study the diffusion of the charged ammonia–copper–ammonia complexes.”
The study was recently published in Nature Communications, and is written by Professor Henrik Grönbeck, Joachim Bjerregaard, doctoral student at the Department of Physics, and Professor Martin Votsmeier, at industrial partner Umicore and Technical University Darmstadt, within the EU-funded project CHASS.
Guarantees high accuracy
Conventional machine learning force fields assume a locality, which does not describe the long-ranged Coulomb interactions that to a large extent determine the properties of zeolite systems. The newly developed machine learning force field is based on extensive first-principles calculations, which guarantees high accuracy.
“Using the machine learning forcefield it has been possible to reveal the atomistic mechanisms for charged ammonia–copper–ammonia diffusion and understand how the composition of the material affects the formation of charged ammonia–copper–ammonia pairs and their stability,” says Joachim Bjerregaard.
The work provides in this way new handles to enhance the performance of the catalytic material for nitrogen oxides reduction, or with extensions for potentially other applications as well, such as one step conversion of carbon dioxide to methanol or longer hydrocarbons.
“The application of accurate machine learning force fields to zeolite systems is an exciting development that helps us to accelerate the understanding of complex systems and suggest new efficient catalytic materials,” says Henrik Grönbeck.

About the research:
The article “Influence of aluminium distribution on the diffusion mechanisms and pairing of [Cu(NH3)2]+ complexes in Cu-CHA” was published in Nature Communications on January 11, 2025, and is written by Joachim Bjerregaard, Martin Votsmeier and Henrik Grönbeck. The work has been funded by the European Union Marie Sklodowska-Curie project CHASS, which connects research groups in academia and industry.
Contact
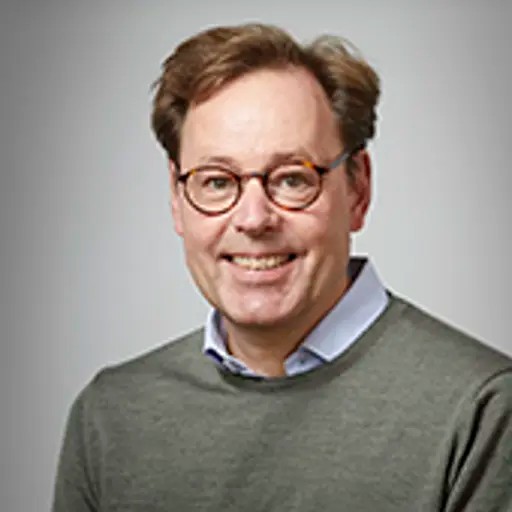
- Full Professor, Chemical Physics, Physics
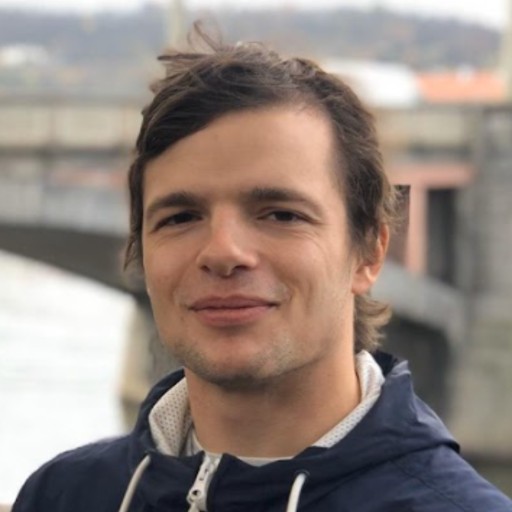
- Doctoral Student, Chemical Physics, Physics