Are you interested in the following questions?
- How can we encode structure (e.g., scientific domain knowledge) into learning systems?
- How does domain knowledge affect uncertainty quantification and out-of-distribution predictions?
- Can these insights enable us to solve problems in a data and computationally-efficient manner?
- How can these strategies help scientific discovery?
Then chances are that you do not want to miss this event!
Overview
- Date:Starts 5 May 2023, 13:00Ends 5 May 2023, 17:00
- Location:Wallenberg Conference Center, Medicinaregatan 20, Gothenburg
- Language:English
- Last sign up date:21 April 2023
At this event we introduce a new CHAIR Research Theme at Chalmers, which focuses on these research questions. The theme is organized by an interdisciplinary team of faculty across Chalmers and GU, and together we aim to stimulate a broad discussion of these research question on campus through a number of social and scholarly activities. Below you find a description of the theme and a preliminary schedule for the event.
Theme description
Modern machine learning and artificial intelligence systems are now sufficiently expressive that we can routinely fit the most complicated datasets with extraordinary precision. Moreover, using classical statistical approaches, we can also estimate in-distribution generalization errors. Nevertheless, fundamental scientific laws of nature equip observed data with structure, and yet many learning systems do not explicitly account for these underlying structures. Consequently, the resulting machine learning models are data-intensive and may generalize poorly to out of distribution data without proper quantification of increased uncertainty.
Within this CHAIR theme, we argue the advantage of considering natural structure when building learning systems. Such an approach has enjoyed success in applications such as image and natural language processing systems and the physical, biological and chemical sciences. Here, we will focus on two broad areas related to the core theme. First, theoretical aspects: data efficiency, out-of-distribution generalization, and uncertainty quantification. Second, applications in science: e.g., inverse problems and surrogate modeling. With this focus, we aim to complement and build on recent advances in the area and build strong international connections to leading labs from the research community. Further, we aim to use the theme to mediate between domain experts in the sciences, machine learning, and AI researchers. Together we anticipate that the theme activities will stimulate research consortia and lead to joint publications and grant applications.
Preliminary Schedule
- 13:00: Arrival.
- 13:30: Introduction to the theme by organizers.
- 13:45: Annika Lang (GU/CTH)
- 14:20: Question and open room discussion
- 14:45: Fika and theme mingle with posters
- 15:50: Ozan Öktem (KTH)
- 16:25: Question and open room discussion
- 16:50-17:00 Closing comments
- 17:00-17:30 Mingle outside
The Structured Learning Theme is organized by Rocío Mercado (CSE), Umberto Picchini (MATH), Moritz Schauer (MATH), Axel Ringh (MATH), and Simon Olsson (CSE).
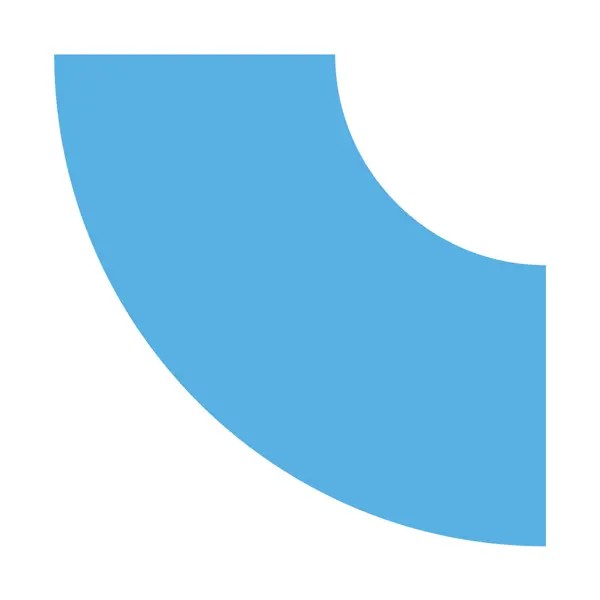
Structured learning
This theme focuses on how to make use of structure in data to build machine learning (ML) and artificial intelligence (AI) systems which are safer, more trustworthy and generalize better. Structure includes the relationship between data, in time and space, and how the predictions change when data is transformed in specific ways, for example rotated or scaled. These topics are abstract and general but have a direct impact on the use of AI and ML in the sciences and in applications such as drugs and materials design, or medical imaging.
Contact
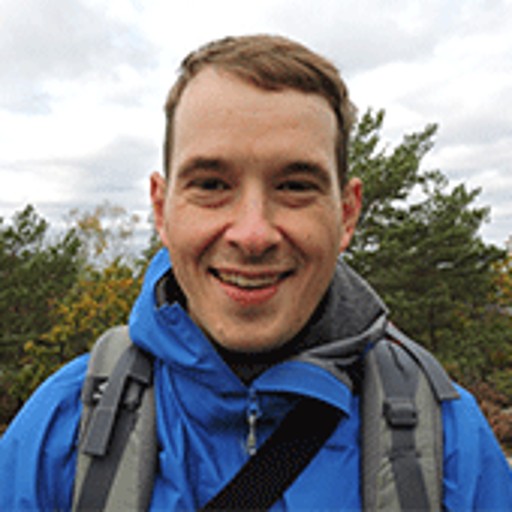
- Assistant Professor, Data Science and AI, Computer Science and Engineering