CHAIR theme event; AI for scientific data analysis
Overview
- Date:Starts 20 April 2023, 13:00Ends 20 April 2023, 15:00
- Location:PJ Seminar Room, building Fysik Origo, Campus Johanneberg
- Language:English
Speakers
Amir Jahangiri and Professor Vladislav Orekhov, Department of Chemistry and Molecular Biology, Swedish NMR Centre, University of Gothenburg.
Abstract
We will give a short intro to recent progress in using AI in NMR spectroscopy, as well as present our novel approach for processing NMR spectra using a deep neural network based on the WaveNet architecture (WNN). Our method is designed to grasp specific patterns over the entire NMR spectra.
If trained on a fixed non-uniform sampling (NUS) schedule, the WNN benefits from pattern recognition of the corresponding PSF pattern produced by each peak. This results in higher quality of the spectrum and more robust reconstruction relative to results produced by the network trained using random NUS schedules.
We demonstrate that the WNN can also successfully perform virtual homo-decoupling in both indirect and direct spectral dimensions. Several other “smart” NMR signal processing will be presented. For example, the WNN can narrow down peaks and improve spectral resolution. Moreover, we demonstrate that our approach often outperforms existing algorithmic methods, and can be used to design new intelligent NMR processing techniques.
Overall, our results highlight the potential of deep learning techniques for improving NMR data analysis.
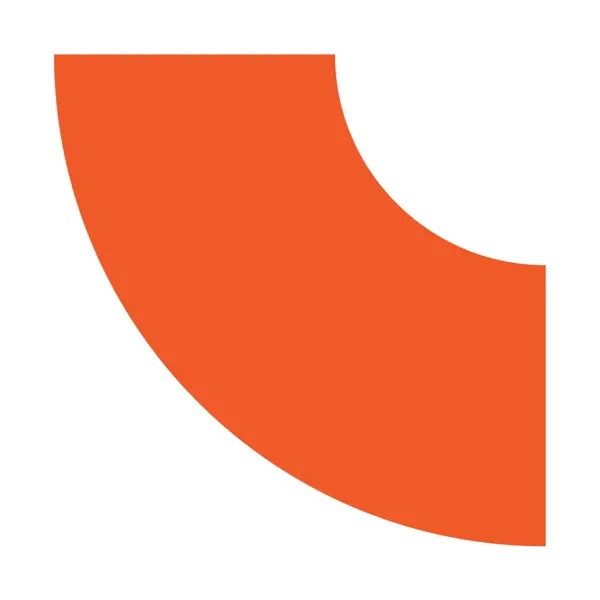
AI for Scientific Data Analysis
This theme is about utilizing the power of AI as a tool for scientific research. AI can be applied to, and potentially speed up, discovery and utilization in a variety of research disciplines, such as microscopy, physics, biology, chemistry, and astronomy.
Contact
- Senior Lecturer, Institution of physics at Gothenburg University