The workshop consists of four sessions spread over three days:
1. Data efficiency and generalization
2. Uncertainty quantification
3. Inverse problems
4. Applications
Overview
- Date:Starts 23 October 2023, 12:00Ends 25 October 2023, 16:00
- Location:
- Language:English
- Last sign up date:29 September 2023
In this workshop we broadly cover topics related to Structured Learning, targeting specifically the following questions:
- How can we encode structure (e.g., scientific domain knowledge) into learning systems?
- How does domain knowledge affect uncertainty quantification and out-of-distribution predictions?
- Can these insights enable us to solve problems in a data and computationally-efficient manner? We will have a particular focus on inverse and surrogate modeling.
- How can these strategies help scientific discovery?
The workshop is organized by the CHAIR theme on Structured Learning: Rocío Mercado (CSE), Umberto Picchini (MATH), Moritz Schauer (MATH), Axel Ringh (MATH), and Simon Olsson (CSE).
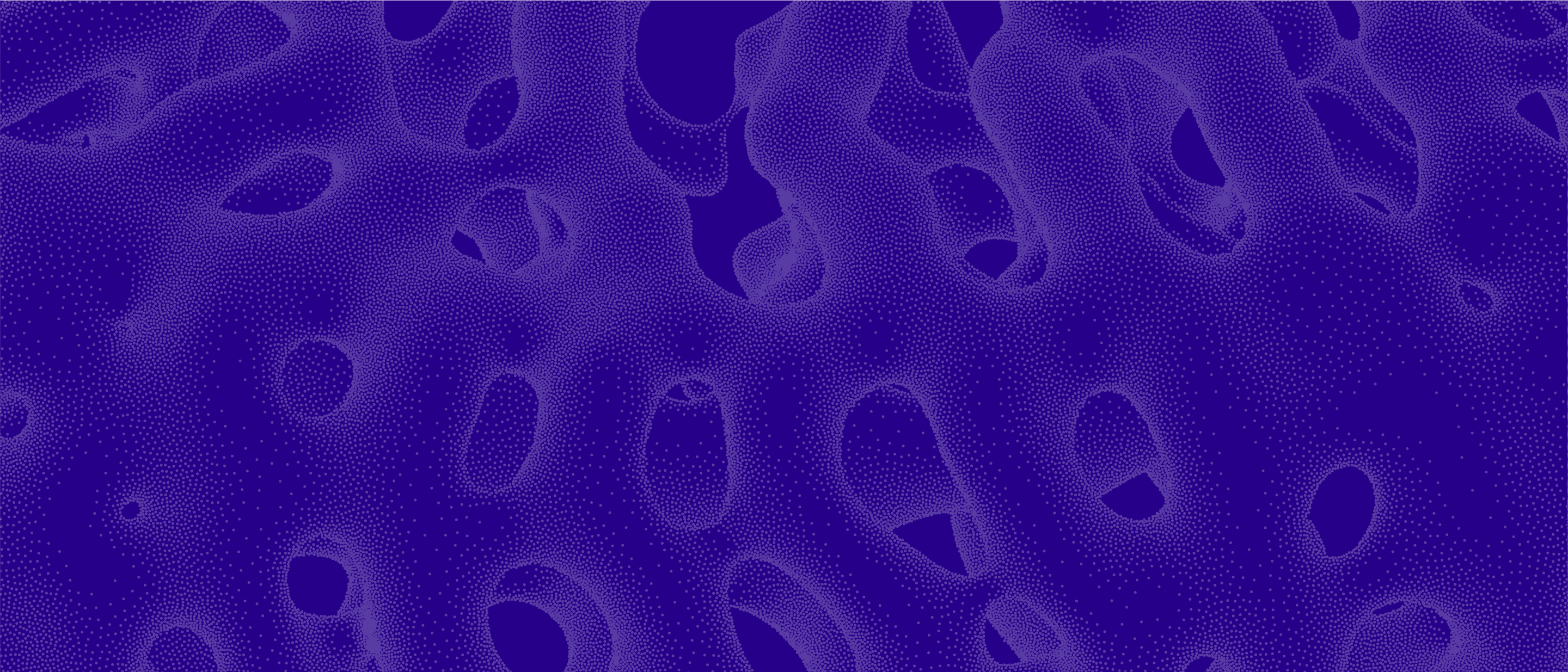
Read more and sign up at the event page
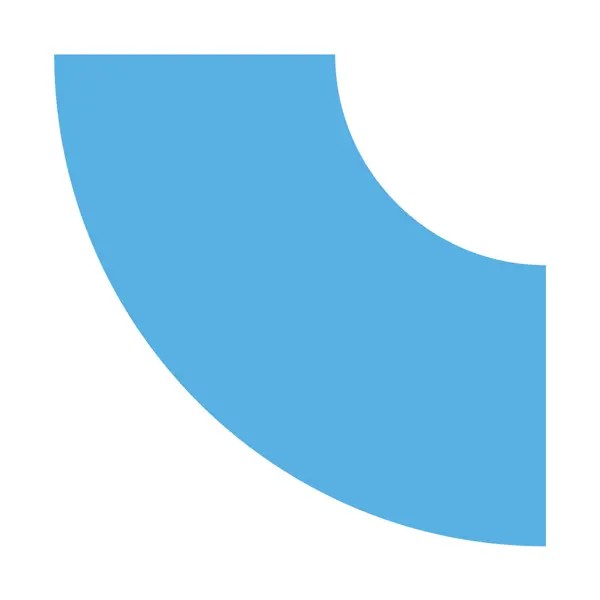
Structured learning
This theme focuses on how to make use of structure in data to build machine learning (ML) and artificial intelligence (AI) systems which are safer, more trustworthy and generalize better. Structure includes the relationship between data, in time and space, and how the predictions change when data is transformed in specific ways, for example rotated or scaled. These topics are abstract and general but have a direct impact on the use of AI and ML in the sciences and in applications such as drugs and materials design, or medical imaging.