Close connection with industry helps ensure that our graduate education remains highly focused and relevant.
Doctoral studies
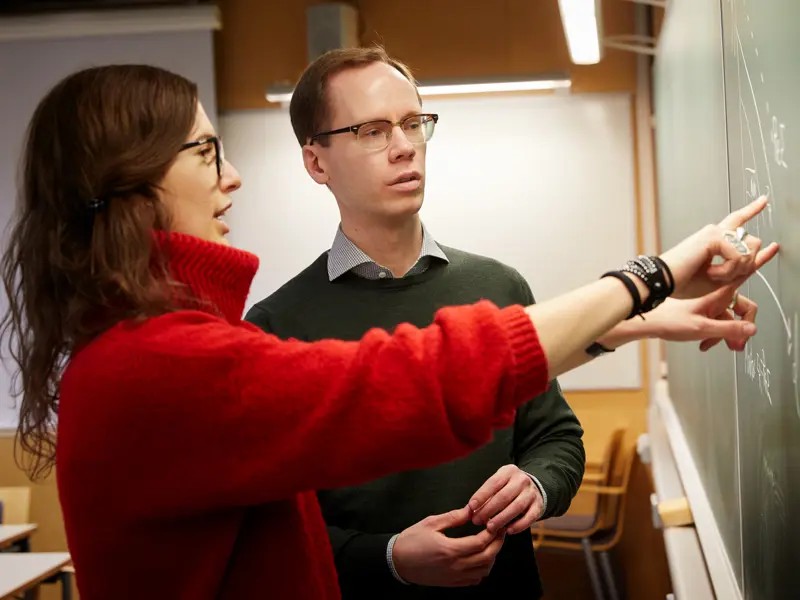
Graduate courses
Doctoral programmes are a combination of individual research, courses and teaching. Doctoral students choose their activities with the help of their supervisors.
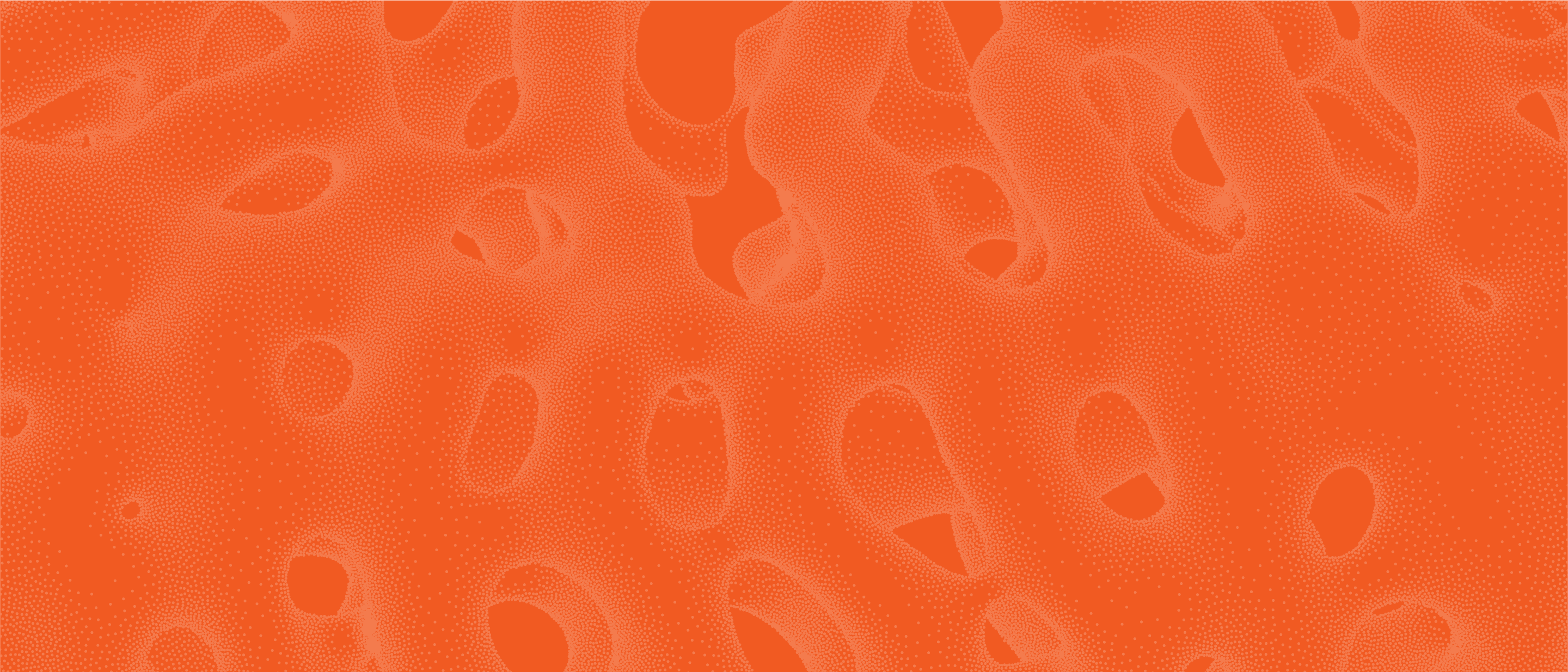
PhD positions
As a doctoral student, you are employed by Chalmers. All doctoral positions are advertised under Vacant positions.
Vacancies