The mathematical sciences are fundamental and indispensable to a large part of modern science and engineering. Progress in other disciplines is often linked to an increased use of mathematics. Mathematics is also a subject in itself, and fundamental research is a necessary condition for its many applications.
The Department is joint for the Chalmers University of Technology and University of Gothenburg.
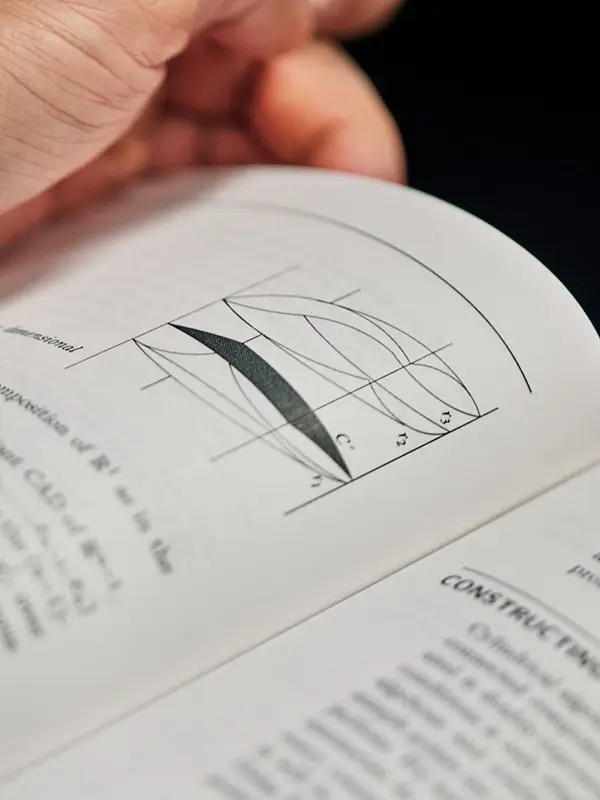
Our research
Read more about current research areas at Mathematical Sciences.
Research at Mathematical Sciences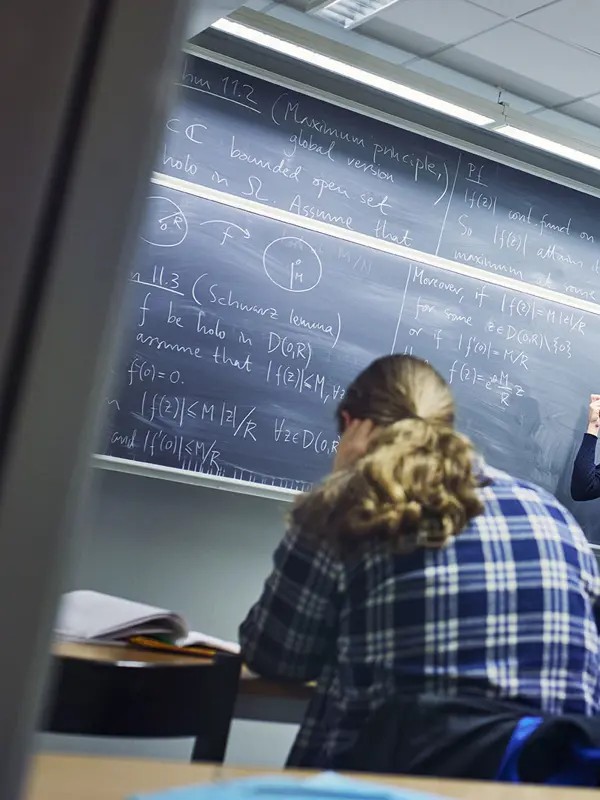
Study mathematics
Read more about studying mathematics in Gothenburg.
Education at Mathematical Sciences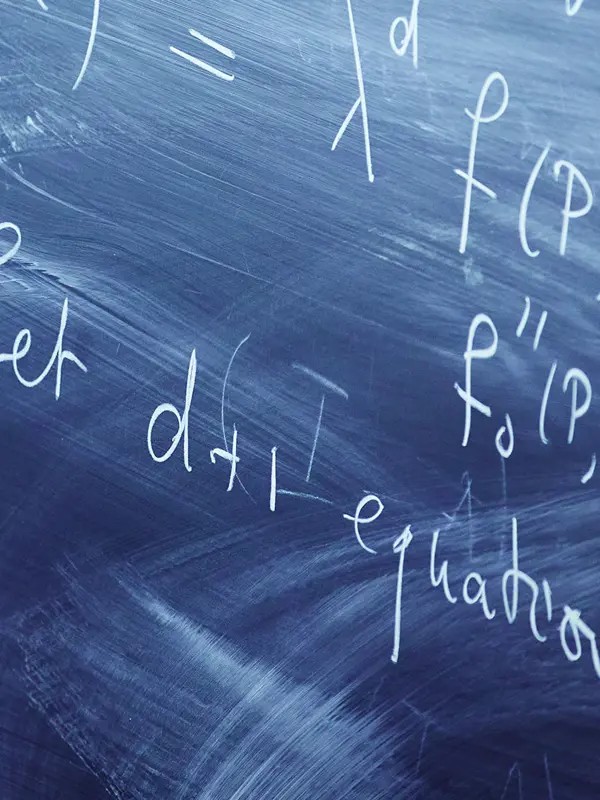
Seminar series
We host recurring seminars within most of our research areas. Follow this link for more information.
Seminar series at Mathematical Sciences